Opinion
How economic uncertainty is pushing financial institutions towards alternative data
Derived from non-traditional sources for financial information, alternative data is becoming an increasingly important tool for investors, in the aftermath of Covid-19, due to a lack of relevant up-to-date data that reflects the current marketplace in real time
12:0816.06.20
Finding data sets that are unique, relevant, and reflect a live snapshot of the true state of the economy is crucial for success in today’s financial markets. In a rapidly changing business environment, alternative data, also known as external data, has become increasingly popular. Alternative data is defined as derived from non-traditional data sources, such as social media networks, giving bankers, investors, and various financial market players information and unique insights to help them evaluate loans, investment opportunities, or business decisions, which are critical in the current uncertain business climate.
Omri Orgad. Photo: Tamara Barelski In the capital markets space, alternative data is viewed as increasingly important. According to a 2019 survey by Greenwich Associates, 95% of trading professionals believe alternative data will become more important to the trading process. Moreover, 85% of banks, investors, and capital markets’ service providers plan to increase spending on data management in the next three to five years, as they increasingly look to it as a source for decision-making insights.
The alternative data market, which is expected to become a $1.7 billion industry in 2020 and double year-over-year, can encompass a range of sources. These include natively digital information, such as web traffic, online buying habits, pricing strategy, social media activity, government publications—for example, pharmaceutical approvals—as well as more granular indicators of financial performance, such as ocean cargo and automobile registration information.
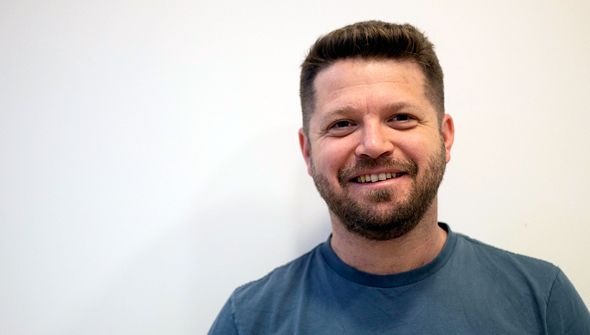
Now, following the coronavirus (Covid-19) pandemic, these numbers are only expected to grow. The reason for this lies in a lack of relevant up-to-date data that reflects the current marketplace in real time. When a bank needs to make a precise and timely decision on the risk ratio of its loan portfolio or whether to provide a loan, it needs to examine all data sources related to that loan. Now, with online data becoming the key source for dynamic data, it is the natural go-to adviser in such cases.
As this new data becomes a powerful differentiator in the search for alpha—the excess return on an investment relative to the market as a whole—a rapidly growing community of buy-side firms are using it to add power to quantitative and fundamental investment models, aiming to outperform the market.
For example, Nasdaq-owned online data marketplace Quandl Inc., which identifies data sets from local firms to build investment models, is partnering with large insurance companies in the U.S. to access insurance policies on new car purchases, enabling users to accurately measure car sales before manufacturers report them. This data is extremely important to, say, hedge fund investors who need investment insights into the auto sector.
Furthermore, in Asia, Nasdaq is building regional-specific data products and is partnering with local fintech companies and other innovative local vendors that have both core data and alternative data that could migrate to Quandl’s platform.
More sophisticated technologies mean we can create datasets that support managers with short-term trading strategies as well as those with a long-term approach, such as institutional investors. An earlier report by Greenwich Associates last year found that 74% of firms surveyed agreed that alternative data is starting to have a big impact on institutional investing, and nearly 30% of quantitative funds attribute at least 20% of their alpha to alternative data.
However, simply finding data is not enough. It can only work if it is properly interpreted and analyzed. By nature, alternative data is harder to consume than traditional financial data; it is often unstructured, does not follow patterns, and is created at a very fast rate. Hence, investors’ growing need for data science talent and new technologies such as automated analytics platforms, testing tools, and fluid data architecture to help them manage all of this data.
Advanced analytics and artificial intelligence technologies, such as machine learning and natural language processing, can be crucial to analyzing data. Machines can process events at roughly 2,000 times the speed of humans, digest vast data sets, and work around the clock without tiring. During the investment process, AI-enabled data collection and processing can increase the volume and quality of idea generation. This increase in data quantities, including alternative data, as well as in computing power, can help investment managers develop a long-lasting competitive advantage.
While some organizations are well on their way to introducing AI-based models, the industry is still in the process of understanding and identifying the operational, regulatory, and technological risks that come with the race for data-driven insights and predictive capabilities. Effective risk-management practices, compliance, and ethical questions will be key to the successful adoption of AI.
Data providers have an opportunity to assist the asset management industry as alternative data and AI appear set to drive the future of investment research. In the near future, we may see active portfolio managers look less to the sell-side for their research needs. Instead, they may develop their own research tools, invest in automated data collection experts and technology, and partner with vendors to supply the intelligence and tools they need. And they need them fast.
Omri Orgad is the managing director of North America at data collection automation company Luminati Networks.