20-Minute Leaders
"It's much quicker to learn from successful people than to make all the mistakes on your own.”
Data science may be a super technical field, but Aviv Ben Arie from Intuit shares with Michael Matias that it’s also strongly about people and business
Click Here For More 20MinuteLeaders
I'd love to start with a little bit about your journey and how you even got introduced to data science.
I began my journey in the cybersecurity space, not as a data scientist, before data science was a very widespread occupation. In the army, I was in a technological unit focused on cybersecurity, and I worked at the Israel prime minister's office.
While working there, I studied computer science and I fell in love with algorithms, research, and data science. I learned that I can combine my two passions and work in the cybersecurity and fraud domain as a data scientist. This is how I got my first data science position at PayPal.
What does "data scientist" mean to you?
A data scientist in the industry is different from what people imagine in academia, researching and creating machine learning algorithms. In the industry, a data scientist spends most of his time working on a specific business problem and deriving a machine learning solution that can have a good business impact.
I received specific tasks to prevent fraud at Intuit or PayPal. I'm in charge of understanding which data I can utilize and how to leverage my vast knowledge of algorithms and machine learning to create a model that ends up in production. My models work in real time every time you hit a login in PayPal or send a transaction.
How has the trend come about to data science in the last 10 or 15 years?
I started looking for my first data science job around six, seven years ago, and there were almost zero positions in Israel. Around four to five years ago, it began to be a position that every company wants to open and hire great data scientists. As a data scientist, I would say that my job gets a bit easier on the technical aspects. A lot of the more advanced machine learning packages, for example, TensorFlow or Keras, didn't even exist when I started. People are looking to study this field more and more. I interview new hires for Intuit, and we have so many applications. Also, universities started opening master degrees in data science specifically.
Are we seeing a similar trend also in terms of people who are entering the workforce and may have to upskill or are looking at what to study now?
Definitely. As one of my volunteering side jobs, I mentor female data scientists who are looking for their next job. I can definitely say that things are getting much more difficult for the candidates. Today, without a master's degree and a thesis in the very specific field of machine learning, it's very difficult to obtain the first job.
People are looking also for experience. I would say that the barriers are getting much higher. But also, I think that there is a lot more training available. My best advice is to gain real work experience even if it's just volunteer work or an open-source project. Have something you can show to prove that you really know how to apply the knowledge to real world problems.
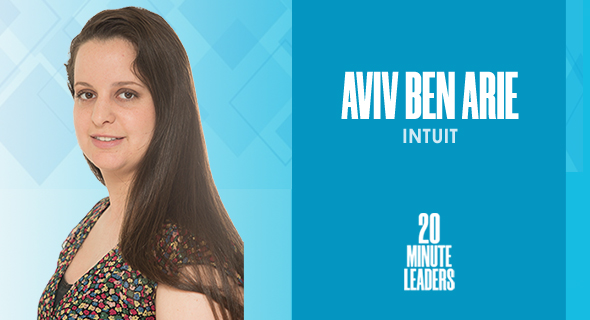
What does the day of a data scientist look like?
In practice, data science is a lot about the business. We're getting paid to create models that make a real impact for our companies. A lot of my day goes into meetings with different stakeholders, connecting all the dots to make sure that the models I’m training are going to solve the business problem in hand. If I create an amazing model in terms of machine learning but the business KPIs don't go up or down as expected, I didn't really do my job.
Around half of my time goes into reading papers and actually writing code, training the models, fine tuning them, writing production code, deploying to production—this is also something that has changed. Now, data scientists are expected to be really well-rounded and be very fluent in coding, deploying to production, and really own the entire pipeline.
You're not just writing math equations. If you don't understand the business needs, you might create a nice model but it's not going to do what the business needs, right?
Exactly. For example, one of the reasons I really love working in the fraud domain is that I have a very rich background in cybersecurity and fraud. I feel that the combination between business knowledge and domain knowledge and my technical capabilities drives better results. I really feel that a strong business sense makes a great data scientist as opposed to just the technical aspects.
If you could talk to Aviv five years ago, what would you tell her is something that is key to your success as a data scientist?
I had no idea how important the connection with the business is. Definitely, get to know the product better. Keep learning and take on different projects. Don't say no to opportunities. Learn from more experienced people; I think this is one of the things that allowed me to advance quickly. I always found mentors and people to learn from. It's much quicker to learn from successful people than to make all the mistakes on your own.
What are the different tools emerging, and where do you think we're going to end up?
I feel that a major concern of people is that auto ML, "Automatic Machine Learning," is gaining a lot of traction. People feel that in 10 years, this profession is going to be redundant. I actually feel that this is a misconception because maybe training a model and deploying it is going to get easier. But business understanding and domain knowledge is not something that you can train an auto ML algorithm to just know. You still need a data scientist that's proficient in cleaning the data, creating features, tuning the model. Also, the human connection: I work very closely with the business and with developers. At the end, everything is all around people.
Well-versed data scientists who know how to handle the business, as well as the technical part, I feel they're going to still have their jobs in 10, 15 years. The velocity of the models we're able to deploy has increased. If in the past it would take me six months to create a new model, today, maybe I can do it in two months. Maybe in five years, I'll be able to do it in two weeks.
What really fascinated you as a kid?
I loved math ever since I could remember myself. One of my greatest realizations is that the world is so vast, and the fact that knowledge is endless and I can just keep on learning, I think this really fascinated me.
What is inspiring you today?
I'm interested, of course, in technology and algorithms. But I would say that what really inspires me is the sense of community. The networking, the mutual help—it really pushed me. I started talking at conferences. This is something I would never have tried without the push of these strong communities.
What did you find so beautiful about math?
It's the only subject I felt that I was really creating something. I can create new problems and solve them. I can think of new things. That's really what inspired me. The fact that it's very productive and it evolves. It's not just something you have to understand, memorize, and go on.
Three words you would use to describe yourself?
Data scientist. I'm a fully trained professional pastry chef. Mentor.
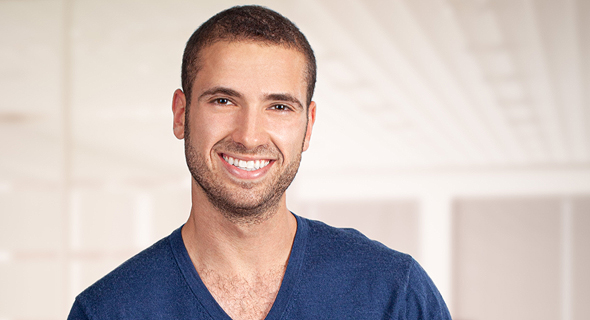
Michael Matias, Forbes 30 Under 30, is the author of Age is Only an Int: Lessons I Learned as a Young Entrepreneur. He studies Artificial Intelligence at Stanford University, while working as a software engineer at Hippo Insurance and as a Senior Associate at J-Ventures. Matias previously served as an officer in the 8200 unit. 20MinuteLeaders is a tech entrepreneurship interview series featuring one-on-one interviews with fascinating founders, innovators and thought leaders sharing their journeys and experiences.
Contributing editors: Michael Matias, Megan Ryan