Opinion
When AI goes too far
“The use of AI to help in the hiring process is growing, and it has found an even broader audience because of the pandemic,” writes Dov Greenbaum
New York’s city council recently passed a bill (38-4) that aims to reign in the use of artificial intelligence (AI) tools in the employee hiring process. Accordingly, the bill would “require that a bias audit be conducted on an automated employment decision tool prior to the use of said tool.” The bill also requires that companies evaluating their employees via AI provide such notice to those applicants. Passed on November 10, the bill has yet to be signed into law, and time is running out with the current mayor Bill De Blasio set to leave office at the end of the year. Even if signed, the law would only come into effect in 2023.
New York is not the only U.S. governmental body interested in this area. Last month, the White House proposed a technology Bill of Rights designed to limit the potential harms associated with artificial intelligence, including technologies like facial recognition that are used in some AI hiring processes.
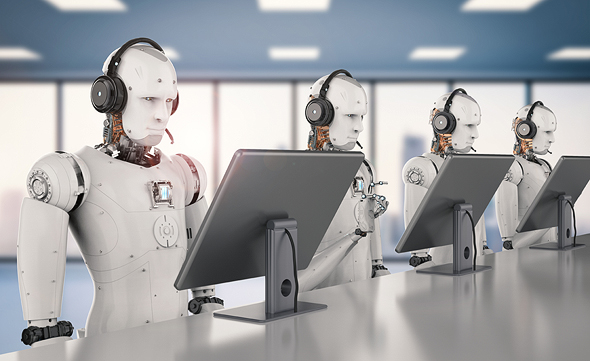
Additionally, the U.S. Equal Employment Opportunity Commission (EEOC) announced an effort to examine the role of AI in employment process, specifically as it relates to the U.S. Federal anti-discrimination laws and regulations. This effort was purportedly in response to a letter from a number of U.S. Senators a year prior.
An often-mentioned area of seemingly clear employment bias underlying much of these recent developments was Amazon’s AI hiring technology that allegedly preferred male candidates; evidently because the data it trained on was mostly male resumes. As they say in computer science: garbage in, garbage out. When the data is biased, it’s likely that the outcomes of the AI analysis will also be biased regardless of the intentions of the AI developers.
All the concerns with the technology notwithstanding, the use of AI to help in the hiring process is growing, and it has found an even broader audience because of the pandemic. With new hires often interviewing only via video chats, the technologies that assess a potential applicant through the analysis of video have become arguably a necessity for employers to competently evaluate a candidate.
However, as the New York city council has correctly assumed, much of these off-the-shelf analysis tools can be biased, even as the tools themselves are sold as a product that can reduce the biases associated with human hiring. The bespoke algorithms are not much better as they also learn the organization’s preferences, which may include various racial, gender, and age biases, and then aim to recreate them in the subsequent hiring process.
More than biases, there are other foundational concerns with AI hiring tools. In 2018, a Harvard, Northeastern and USC joint study found that algorithms often overestimate the value of various features of a potential employee once they are interviewed, perhaps even resulting in non-sensical hiring decisions.
These AI hiring algorithms have already gotten their developers into trouble. In 2019, the Electronic Privacy Information Center (EPIC) filed a complaint with the US Federal Trade Commission (FTC), regarding the algorithms used by HireVue, a company broadly employed by corporations ranging from retailers like Target and Ikea, to multinational banks like JP Morgan and Goldman Sachs. HireVue’s algorithms have a huge impact on the employment process: In 2020, the company claimed to have interviewed nearly 6 million applicants. More recently, in October 2021, it further claimed to have interviewed over a million applicants in a single month.
In their complaint, EPIC argued that HireVue supposedly employs facial recognition algorithms to assess applicants, although the complaint seems limited to semantics as HireVue acknowledges that it uses facial expressions and facial movements in its analysis.
Nevertheless, HireVue, under fire from other groups as well, and likely given the growing global backlash against facial recognition technologies has begun to phase out the facial recognition aspects of its software.
Given their general use of AI in their analysis of potential hires, watchdog groups have argued that companies like HireVue should be more accountable and transparent and that they should do more to ensure their accuracy, reliability and validity, as per the Universal Guidelines for Artificial Intelligence (UGAI) and the OECD’s AI guidelines. To its credit in response to the New York bill, HireVue wrote that it welcomes any legislation that demands that all vendors meet the high standards that HireVue has supported since the beginning.”
While there has been some praise for New York’s efforts, others claim that it does not go far enough in combating AI bias, and in fact, could provide legal cover for companies that can pass the bias audits but are nevertheless potentially employing problematic algorithms. Some have even suggested that when AI algorithms impact issues like health, housing and careers, then there always ought to be a human in the loop.
Notably, biases exist even when the analysis of an applicant is conducted through non-AI algorithms as well. To wit, in the field of legal academia, Fred Shapiro, a law librarian and lecturer at Yale Law School just published his analysis of the most cited legal scholars. This is not Shapiro’s first foray into this space; his papers on the subject are highly respected. Many law professors publicly tout their inclusion on these lists.
However, like biases in the aforementioned AI algorithms, these compiled lists perpetuate deep-seated and arguably anachronistic philosophies of what ought to be the scope of scholarship for legal academics. Consider, for example a legal scholar with both an extensive publication record in law as well as in another field. Such a scholar might not be included in Shapiro’s lists, regardless of how many thousands of citations they have. As such, these transdisciplinary researchers might be excluded from the recognition such lists provide with regard to the employment and promotion processes in legal academia.
- Are smart contracts actual contracts?
- Irrational NFT exuberance requires new IP law
- Real World Data key to drug discovery, reducing carbon footprint of clinical trials
Succinctly, the biases represented in Shapiro’s analysis suggest that a multidisciplinary legal scholar’s relevance to the legal community is limited to just the narrow scope of their law review articles; all their other scholarship, regardless of its quality or association with their overall research record, is irrelevant. To add insult to injury, the legal academia has even long recognized blogging as a valued component of a researcher’s scholarship and as a valued asset to their institution, but seemingly not yet publications in other fields.
The legal issues raised by emerging technologies such as the concerns discussed above with regard to AI algorithms in the hiring process will likely mean that more legal academics will have scientific and technical training and perhaps even extensive scientific publishing records. Ironically, the same legal academia that now wants this cross-disciplinary scholarship will likely continue to be biased against those who provide it, at least with regard to the value of much of their research.
Prof. Dov Greenbaum is the director of the Zvi Meitar Institute for Legal Implications of Emerging Technologies at the Harry Radzyner Law School, at Reichman University