Interview
Mobileye's Mathematical Approach is the Key to the Autonomous Vehicle Revolution, Says Chief Technology Officer
A leader in autonomous driving systems, Mobileye sees the future of car ethics in Mathematics
2019 is here, and autonomous cars are still a thing of the future. While a few years ago the world seemed on the brink of a driverless car revolution, there is now growing talk among critics and artificial intelligence experts alike about a technological bottleneck that could mean a truly autonomous car is years if not decades away. Crashes, like the one in which Uber's test car killed a pedestrian in 2018, are adding fuel to the fire.
For daily updates, subscribe to our newsletter by clicking here.
While some of the major players in the industry reject the criticism, pack leader Mobileye does not. What’s more, the company claims it already has a solution—the Responsibility Sensitive Safety (RSS) system, a mathematical model outlined in a 2017 paper by co-founder and CEO Amnon Shashua and chief technology officer Shai Shalev-Shwartz. Mobileye believes the model will help it meet the objective of launching an autonomous car at the beginning of the next decade.
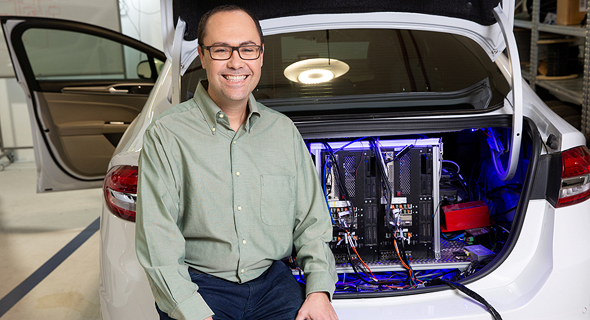
Mobileye and parent company Intel are very familiar with AI, deep learning, and big data technologies, and make use of such algorithms across a variety of products and projects, Shalev-Shwartz said in a December interview with Calcalist. But while deep learning is the basis for a lot of new abilities, it is not a perfect solution, and it is not the answer to every problem in the world, he explained.
Deep learning, at its heart, is a technology used to teach a computer to identify patterns based on a database of examples, such as a series of road images with and without pedestrians. Specific algorithms leverage the technology to perform actions, like recognizing a pedestrian in an image the computer has never seen before.
The problem, according to Shalev-Shwartz, is that the output of such systems is right, on average. "If you ask the neuron network whether the new image displays a pedestrian, it will get the answer right, on average. It could be right in 99% of cases, or even in 99.9% of cases, but an average-based behavior will never be 100% right, that's just the limits of the technology," he said.
And with autonomous cars, you cannot settle for anything less than 100%.
Another criticism of deep learning systems is that when it comes to their decision-making process, they are a black box. As Shalev-Shwartz points out, if autonomous cars are to be accepted on regular roads, the public wants to know just how that car is making its decisions, and what rules it follows.
"Currently, that's beyond the capabilities of deep learning," Shalev-Shwartz said.
Mobileye is instead banking on an AI model that uses mathematical proofs instead of deep learning. The model takes human notions such as what it means to be a careful driver, or what constitutes a dangerous situation on the road, and turns them into a mathematical equation.
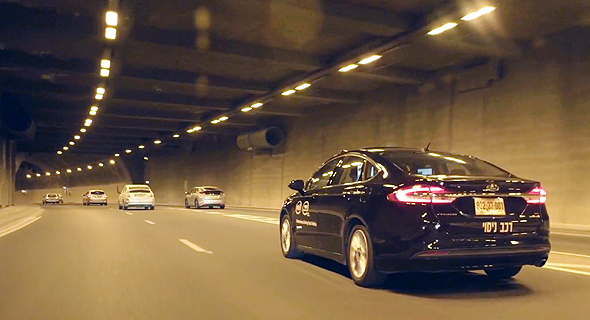
To create the model, the company defined a series of dangerous situations, the factors that come into play, and the relevant variables, and created a system of rules and equations. Those were then checked against a U.S. database of six million police reports of accidents.
The final model uses five rules, each with its relevant equations.
"The first rule is common sense—don't ram someone from behind," Shalev-Shwartz explained. The rule needs to be defined very specifically because it is not always true, he said. In a normal situation, the responsibility of keeping distance lies on the rear car, but if you are standing in place and the driver in front of you reverses and rams into you, for example, technically you still rear-ended someone.
To formulate the equation, Mobileye took the worst case scenario, in which the front car brakes abruptly, and created a formula that specifies that if the distance between the cars is larger than x, they'll come to a stop without hitting each other, but if the distance is smaller, the crash is inevitable. The formula also establishes the behavior of the rear car.
"Newton's laws of motion dictate how both cars move until they come to a complete stop," Shalev-Shwartz explained. Therefore, Mobileye can build an equation that determines how to prevent the rear car from ever rear-ending another. As it’s a mathematical formula, it's something people can examine and understand, unlike the decision-making process of a deep learning system, he pointed out.
The other four rules dictate how to bypass another vehicle, how to determine the right of way, how to behave in areas with a limited field of view, and the responsibly of preventing an accident in a dangerous situation.
In terms of the technology itself, Shalev-Shwartz thinks companies could keep to the previously discussed deadlines. Mobileye, he points out, intends to start piloting an autonomous robot taxi in Tel Aviv in 2019. But public acceptance always affects technology in its last stages, especially in matters pertaining to safety, he said.
- Intel, Mobileye, Volkswagen Team on Israeli Self-Driving Taxi Venture
- Safety Is a Priority with the Autonomous Vehicle, Says Mobileye Vice President
- Baidu Teams Up with Intel’s Mobileye to Make Self-Driving Cars Safer
When the technology is ready, it will start to trickle in, and then become more commonplace as people accept it and trust it. Transparency will play a large part in that acceptance, he added.
"If the revolution fails to come, it won't be an issue of technology," he said.