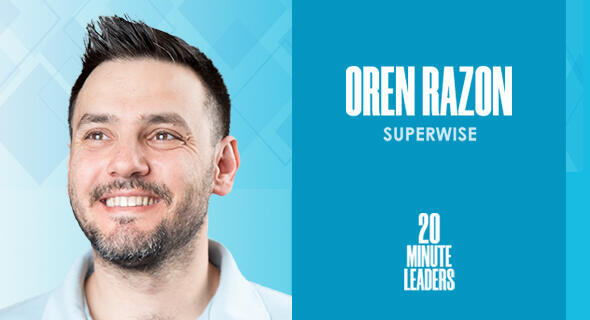
20-Minute Leaders
“It's not enough to understand that AI is functioning. You really need to understand whether it's working as expected.”
Bridging the gap between leveraging AI and trusting the technology is the mission of Superwise.ai, says Oren Razon, co-founder and CEO.
Bridging the gap between leveraging AI and trusting the technology is the mission of Superwise.ai, says Oren Razon, co-founder and CEO. He saw that many companies were excited to develop AI solutions, but people were more hesitant to actually deploy them. He explains that it is crucial to know that software is working properly when you are going to trust it to drive your business. Razon says for AI, it isn’t enough to know the algorithms are functioning; you really need to know if they are working as you expected. He shares that if something in the world or your business changes, your algorithm can still work, but the quality of its decisions may be low, something you may not discover until much later. He shares that Superwise provides the solution with model observability and troubleshooting to help reduce the risks of using AI.
Oren, I'm excited to talk to you about your own background, Superwise.ai, and this greater challenge that we're dealing with.
I'm always saying that I've been doing Superwise for the last 17 years. Because as a software engineer that started at Intel, we had a small group of people that thought that the idea of doing something with data mining on top of data could be interesting. Quite fast, we saw that we could do quite amazing stuff and we could actually solve strategic stuff for Intel. It was like being a small startup inside a giant like Intel.
Then I decided to go outside and to help other companies to achieve the same. I started my own data science as a service company, helping other companies to start in and embrace AI and start to put it in real life. Nobody really knew how to do that. I've helped other companies to do the research and to do the heavy lifting. AI is really becoming a core in any core business operation. From the trenches, I saw that whenever it comes to production and you really need to use it, everybody starts to hesitate. "Wait a minute, will I let this black box drive my business?" After seeing that over and over, I decided to found Superwise with the mission to help to bridge the gap between the technology, the ability to use AI, and the ability to really trust it and to bring the optimal results to the business without the risks.
Talk to me about the risks. When I use software, I don't necessarily know what's happening in the backend, but I know that it works because others are using it. What is the difference between that and using an out of the box algorithm in machine learning?
I think the analogy for other software applications is great. If you ask an engineer on the street if he will deploy something to production without putting some kind of monitoring, he will tell you, "Hell no. I must have something to make sure that the CPU is okay, that the memory is okay." Whenever we let software drive our business, we want to be sure that it's working as expected.
We're seeing more and more companies that want to embrace AI, want to do that faster, in a much more standard way, and still have full confidence that it will work as expected. We see the need for model observability. It's not enough to understand that it's working from a functional perspective. You really need to understand whether it's working as expected.
For example, if I'm a bank and I was using a basic rule engine to decide whether to approve or to reject a loan, it was very simple for me to understand whether it's working. But now, when the algorithm is leveraging so many data points, there is the potential of using and leveraging the data, but it comes with the risk. It means that you are totally dependent on your data. So if something would start to change, the behavior of the external world or maybe even something internally, the algorithm will still work from a functional perspective but the quality of his decisions will be very low. The problematic thing about it is that you won't know until it's too late. Algorithms are doing what we want them to do. They are predicting stuff that will be discovered later on. They will approve the loans, and after a few months, you will start to understand that your business was impacted from approving a lot of loans that shouldn't have been approved.
But we have so many new challenges around using AI because it's not only about the business performance, it's also about brand risk and biases. There’s compliance; there are so many new risks that come with AI. Superwise is here to solve them and to let organizations scale effectively while reducing those risks.
Tell me about Superwise. How do you tackle this problem of observability?
Looking at the ecosystem of developing machine learning applications and embracing AI at high scale in enterprises, we see that companies use all kinds of different platforms to develop the algorithms. We know how to provide model observability as a service. We come with our solution, and we know how to integrate to any platform. You still have the ability to deploy your machine learning in a way that you want to deploy it. Once you connect to Superwise, immediately, you get eyes into production. You understand what is the performance of your machine learning-based process, the level of quality of data that comes in, the level of risk that your algorithm is exposed to, what biases are occurring.
A key ability in Superwise is to understand all those things that go under the radar. We know to pinpoint and to say, "For specific subpopulations in a specific area in the United States that has a specific demographic, your algorithm is now underperforming." We give them the visibility in a super high resolution and also the automation around it to reduce the level of noise and to be able to detect those issues as they occur. Then we lead them through the troubleshooting and resolution process until it's been fixed.
Why is this the right time for Superwise.ai to grow?
I think it's great timing because the problem is already there, the market is already very big. You can see so many companies, especially enterprises, that already leverage AI inside their core business operation. But it's still an emerging growing market that is growing exponentially every day. It's a great environment for us as a startup to build ourselves, to build a product, to work with our customers closely to make sure that we are building the right product for this market, and to become the standard tool for any company that leverages AI.
Perhaps the people that are looking at the insights from Superwise aren’t necessarily the machine learning engineers. They can be data analysts or even on other functional teams. Right?
The number of stakeholders that are being involved in the process of model observability has an amazing potential. Right now, mostly we're talking about the technology teams that are still owning the process. We started to experience it ourselves with our customers; we see that the risk analyst, the marketing analyst, everybody from the business starts to say, "If you know about something that is misbehaving, which means that our business is underperforming, I want to know about it." We are monitoring not only the black box from the technical perspective, we are actually monitoring the business process. There are so many potential stakeholders. They need to be aware of it, and they want to consume our insights.
What are the ways you're measuring the success of this platform?
The main KPI is to alert about stuff that’s happening. The way to measure it is actually to reduce the time to detect and fix issues. Instead of detecting it and fixing it after three months, doing that after one day.
But then, come the secondary KPIs. One of the things that could kill your value proposition is alert fatigue. A very important KPI for us is not only to show that we are able to capture those issues, but we can do that with a very low false positive rate. The second thing is the ability to develop new algorithms is really an iterative task. We see that data scientists are starting to use Superwise to start and plan their next iteration of model development.
Michael Matias, Forbes 30 Under 30, is the author of Age is Only an Int: Lessons I Learned as a Young Entrepreneur. He studies Artificial Intelligence at Stanford University, is a Venture Partner at J-Ventures and was an engineer at Hippo Insurance. Matias previously served as an officer in the 8200 unit. 20MinuteLeaders is a tech entrepreneurship interview series featuring one-on-one interviews with fascinating founders, innovators and thought leaders sharing their journeys and experiences.
Contributing editors: Michael Matias, Megan Ryan